Abstract
We analyze a large dataset of fully employed individuals gathered through incentive-compatible surveys to study who joins professional social network sites (SNS) such as LinkedIn. We test the conflicting predictions that: (a) individuals who are unsatisfied with their career status adversely select into professional SNS to reap marginal online network benefits, versus (b) high-status individuals positively select into these networks because they are more likely to receive invitations to join. Our tests support (b) and reject (a). Similar estimations for private SNS (e.g., Facebook) reveal that the observed effects are specific to professional SNS and are not driven by unobserved differences in social capital or the affinity to use social media. We also find that environments conducive to professional social interactions increase the likelihood of using professional SNS. Controlling for sample selection bias does not qualitatively change the results.
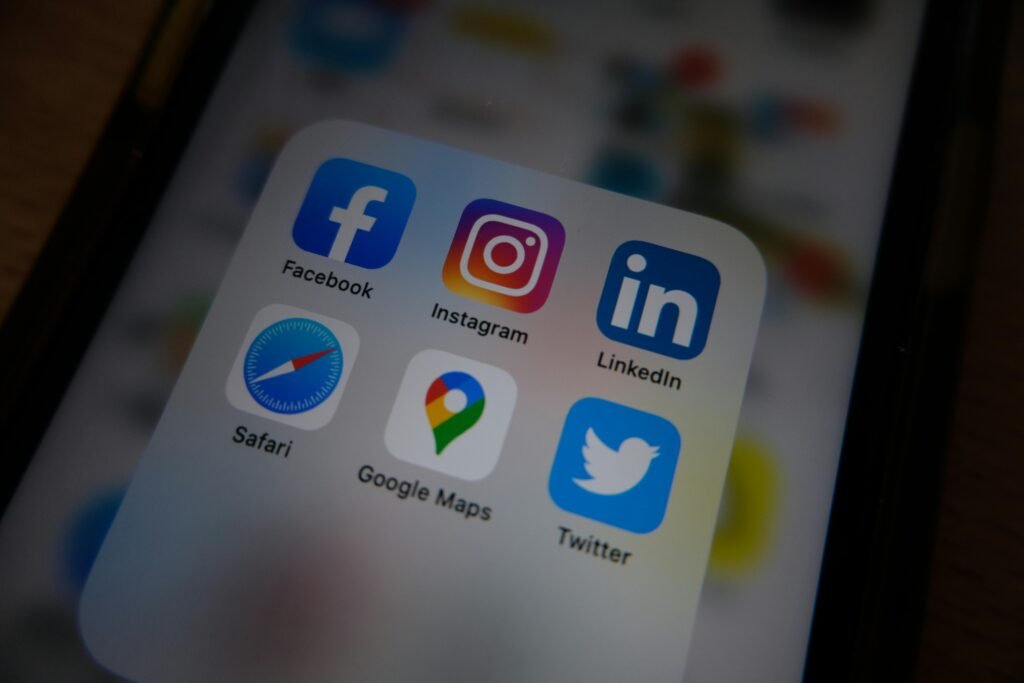
Abstract
- We analyze a large data set of fully employed individuals gathered through incentive-compatible surveys to study who joins professional social network sites (SNS) such as LinkedIn:
- We analyze: This indicates that the authors conducted a detailed examination or study.
- A large data set of fully employed individuals: The study focuses on a significant number of people who are all employed full-time.
- Gathered through incentive-compatible surveys: The data was collected using surveys that likely offered incentives for participation, ensuring honest and reliable responses.
- To study who joins professional social network sites (SNS) such as LinkedIn: The aim is to understand the characteristics and motivations of individuals who become members of professional networking platforms like LinkedIn.
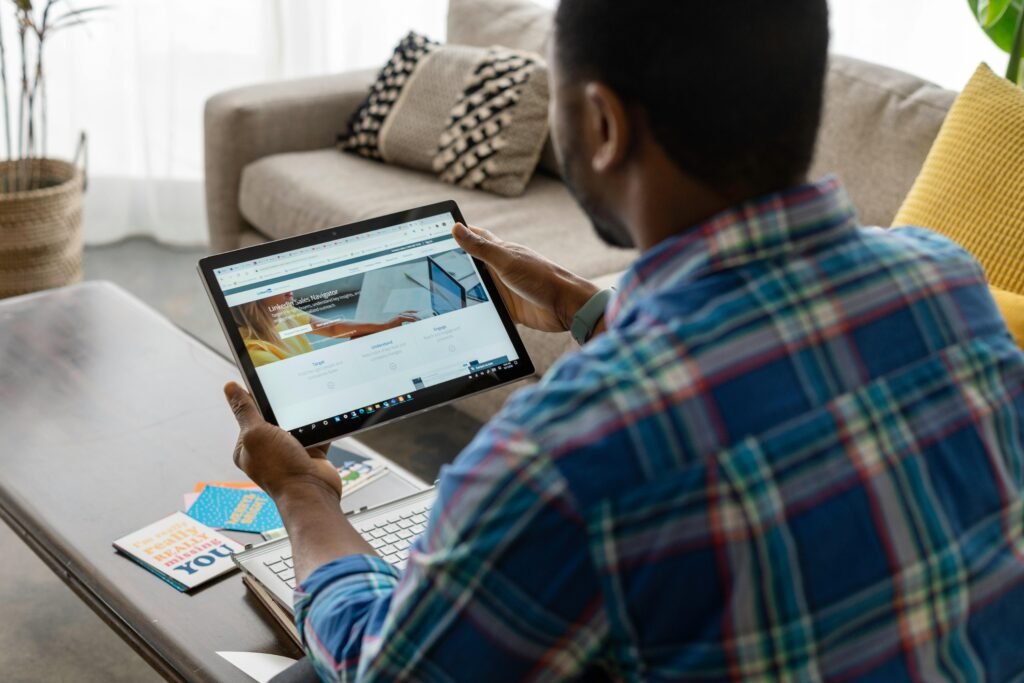
- We test the conflicting predictions that:
- a) Individuals who are unsatisfied with their career status adversely select into professional SNS in order to reap marginal online network benefits:
- Individuals who are unsatisfied with their career status: This refers to people who are unhappy or dissatisfied with their current job or career progress.
- Adversely select into professional SNS: These individuals might join professional networking sites because they are looking for new opportunities or connections to improve their career.
- In order to reap marginal online network benefits: They are hoping to gain small but potentially significant benefits from networking online, such as finding new job opportunities or professional advice.
- b) High-status individuals positively select into these networks because they are more likely to receive invitations to join:
- High-status individuals: This refers to people who are already successful or hold prestigious positions in their careers.
- Positively select into these networks: These individuals join professional networking sites because of their high status.
- Because they are more likely to receive invitations to join: They are often invited to join these platforms due to their professional reputation or status.
- Our tests support (b) and reject (a):
- The study’s findings indicate that high-status individuals are more likely to join professional SNS, and the hypothesis that dissatisfied individuals join for marginal benefits is not supported.
- Similar estimations for private SNS (e.g., Facebook) reveal that the observed effects are specific to professional SNS and hence not driven by unobserved differences in social capital or the affinity to use social media:
- Similar estimations for private SNS (e.g., Facebook): The authors also looked at data from social networks that are not professional, like Facebook.
- Reveal that the observed effects are specific to professional SNS: The findings that high-status individuals are more likely to join are unique to professional sites like LinkedIn.
- And hence not driven by unobserved differences in social capital or the affinity to use social media: This suggests that the results are not influenced by other factors like general social connectivity or the inclination to use social media.
- We also detect that environments conducive of professional social interactions increase the likelihood to use professional SNS:
- Environments conducive of professional social interactions: Settings or situations that encourage professional networking and interactions.
- Increase the likelihood to use professional SNS: People in these environments are more likely to join and use professional networking sites.
- Controlling for sample selection bias does not qualitatively change the results:
- Controlling for sample selection bias: The authors accounted for potential biases in how the sample was chosen.
- Does not qualitatively change the results: The main findings remain the same even after adjusting for this bias, indicating the robustness of the results.
Introduction:
- Few markets have remained unscathed from the transforming forces of the internet.
- Almost all industries have been changed by the internet. Very few markets (or industries) have not been affected by the changes brought by the internet. The internet has significantly transformed how most businesses operate, sell products, and connect with customers. For example, online shopping has changed the retail market, and streaming services have changed the entertainment industry. However, there are still a few areas that haven’t been impacted as much by the internet’s influence.
- Evidence of this phenomenon includes markets for advertising (Levin and Milgrom 2010), insurances (Brown and Goolsbee 2002), consumer products (Kamenica, Mullainathan, and Thaler 2011; Baye and Morgan 2001), and dating (Hitsch, Hortaçsu, and Ariely 2010).
- We can see the internet’s impact in advertising, insurance, consumer products, and dating.
- Labor markets are likely to be added to this list, given the great potential of the internet to reduce the search frictions that typically characterise job matching processes (Stigler 1962; Mortensen 1970; Mortensen, and Pissarides 1994; Diamond 1971; Shimer, and Smith 2000).
- The job market will probably be added to this list because the internet can make it easier to find jobs and match employers with employees.
- Alleviating job search frictions may indeed have a material impact on wages and other career outcomes (Van Den Berg, and Van Vuuren 2010; Gautier, and Teulings 2006).
- Making job searches easier could affect salaries and other job-related results.
- Surprisingly, studies fail to consistently establish a link between internet-based job-matching and employment outcomes.
- However, research has not always shown a clear connection between online job searching and employment results.
- Whereas Kuhn, and Skuterud (2004), Stevenson (2008), and Kroft and Pope (2014) find no internet-related effects on job matching quality, unemployment duration or the rate of unemployment, only Kuhn, and Mansour (2014) report that internet-based job search is associated with significantly shorter unemployment spells.
- Some studies (by Kuhn and Skuterud, Stevenson, and Kroft and Pope) found no internet impact on job matching, how long people are unemployed, or the unemployment rate. But Kuhn and Mansour found that online job searches can help people find jobs faster.
- One potential reason for the lack of consistent evidence of the internet’s impact on employment outcomes is the fact that previous studies ignored the important role that social networks play for matching on the labour market.
- One reason why studies don’t always show the internet’s effect on jobs might be because they didn’t consider how important social networks are in finding jobs.
- Research on the role of social networks on job matching documents that around half of all jobs is allocated through social contacts (see surveys in Ioannides and Loury 2004; Topa 2011; Galenianos 2014).
- Studies show that about half of all jobs are found through personal connections.
- Recently, online platforms such as LinkedIn emerged in the professional realm that help users to manage business contacts and to facilitate communication within the networks these contacts span.
- Recently, websites like LinkedIn have appeared, helping people manage business contacts and communicate within their networks.
- Hence, professional social network sites (henceforth referred to as professional SNS) may have profound implications on the scale and content of career-related information exchange, potentially resulting in an increase in the importance of social network effects in labor markets.
- Therefore, these professional social networking sites could greatly affect how career information is shared, making social networks even more important in the job market.
- As a first step to assess the question of how social online networks influence the labor market, we examine who is more likely to become a user of professional SNS.
- To understand how these online social networks impact the job market, the first thing we look at is who is more likely to use professional networking sites like LinkedIn.
- Selection into social online networks may essentially be driven by two forces: (1) the individual’s motivation to join a network, and (2) the motivation of existing platform users in the individual’s social network to invite her.
- People join online social networks because of two main reasons: (1) they want to join, and (2) their friends or colleagues on the network invite them.
- Provided that individuals expect career-related benefits such as higher wages and other beneficial outcomes from their membership at professional SNS, the former mechanism may give rise to adverse selection of those who are currently unsatisfied with their pay and career status.
- If people think they will get better jobs or higher pay by joining, those who are unhappy with their current jobs and salaries are more likely to join.
- Adverse selection may also occur due to individuals aiming to overcome offline constraints to build a larger social network because of the low number of potential contacts in their residential or organizational environment.
- People might join because they want to expand their network, especially if they don’t have many contacts in their current location or workplace.
- The latter mechanism is likely to give rise to positive selection into professional SNS, because individuals with a higher “network value” to others or individuals in an environment providing a rich set of social contacts should receive more invitations to join the platform.
- When friends or colleagues invite someone to join, it often happens because that person is valuable to the network or already has many connections, leading to positive selection.
- Receiving an invitation by existing platform members has been shown to be instrumental for whether or not an individual adopts such a new service (e.g. Katona, Zubcsek, and Sarvary 2011).
- Invitations from friends or colleagues are very important in deciding whether someone will join a new online network.
- As a result of these conflicting predictions, it becomes an empirical question of whether new social network platforms are subject to adverse or positive selection.
- Because of these different reasons for joining, it’s a question for researchers to find out if new online networks mostly attract unhappy individuals (adverse selection) or well-connected and valuable individuals (positive selection).
- We use a large data set generated through online surveys conducted on the website of the top-selling German weekly news magazine Der Spiegel – Spiegel Online.
- We used a big data set from online surveys done on the website of Der Spiegel, a popular German news magazine.
- Survey participants were asked about a range of job-related issues such as pay and pay satisfaction, employer size, and industry.
- The people who took the survey were asked questions about their jobs, like how much they earn, if they are happy with their pay, the size of their employer, and the industry they work in.
- Further, they were requested to provide information on the location of residence, and education.
- They were also asked to give information about where they live and their education.
- The sample is of particular interest in the context of our study because it contains information about the participants’ membership at Xing and LinkedIn, which are Germany’s two dominant professional online networks.
- This data is especially interesting for our study because it includes whether the participants are members of Xing and LinkedIn, the two main professional online networks in Germany.
- Participation in the survey was not remunerated, but after filling in the survey, every participant received a report on the average salary of an employee with her characteristics and credentials.
- People did not get paid for taking the survey, but after completing it, they got a report showing the average salary for someone with their qualifications and background.
- Hence, the survey was incentive-compatible in the sense that only by truthfully reporting the individual received a useful feedback.
- This means that the survey encouraged people to give honest answers because only then would they get useful feedback about their salary.
Explanation Line by Line in Simple Language:
- We find strong evidence in support of positive sorting and against adverse selection into professional online networks.
- We found clear proof that people who join professional online networks are usually well-qualified and successful, not those who are unhappy with their jobs.
- Educational achievements, the position in the managerial hierarchy, current residual pay, pay satisfaction, and expected pay increases in the next five years are all positively related to membership at LinkedIn and Xing.
- People with good education, high job positions, higher current pay, satisfaction with their pay, and expectations of pay raises are more likely to be on LinkedIn and Xing.
- Moreover, it seems that individuals who are exposed to environments that facilitate forming social relationships in the offline world, such as in large cities and organizations, are also more likely to join professional SNS.
- Also, people who live in big cities or work in large organizations, where it’s easier to meet people and make connections, are more likely to join professional networks.
- One may argue that some of the listed effects are due to spurious correlation, for example, because individuals working at larger firms are more likely to use social online media of all kinds.
- Some might say these results are misleading and just happen because people at big companies use all kinds of social media more.
- To rule out this alternative explanation, we estimated similar models for the most popular private online networks such as Facebook.
- To check this, we looked at similar data for private social networks like Facebook.
- If membership was not due to economic factors such as career-related benefits, one may expect to observe similar effects across both sets of platforms.
- If joining was just because people use social media a lot, we should see the same pattern on Facebook.
- Indeed, we find no similar patterns of positive selection into private online networks such as Facebook.
- But we did not find the same results for private networks like Facebook.
- Finally, addressing the non-response bias does not qualitatively alter our results.
- Even after considering that some people might not have responded to the survey, our main findings remain the same.
Explanation Line by Line in Simple Language:
- Our paper contributes to the literature in multiple ways.
- Our research adds valuable information to existing studies in several ways.
- First, we extend empirical research on social networks by shedding light on how individuals sort into professional online networks.
- First, we expand studies on social networks by explaining how people join professional online networks.
- While there are a few papers that study adoption patterns of private SNSs such as Facebook (e.g. Ellison, Steinfield, and Lampe 2007; Ellison et al. 2014), professional SNS have received scant attention by researchers, even though they recently became widely popular (e.g. McLarty and Rosen 2013).
- Although some studies look at how people use private social networks like Facebook, there hasn’t been much research on professional networks, even though they’ve become very popular recently.
- In contrast to previous studies that examined the influence of personality traits such as overtness and conscientiousness (McLarty and Rosen 2013), our focus is on the complementary issue of adverse and positive selection into professional online networks along socio-economic dimensions.
- Unlike previous studies that looked at personality traits, we focus on whether people who join professional networks are already successful or disadvantaged based on their socio-economic status.
- Thus, one of the main implications of this result is that online platforms may contribute to a redistribution of social network benefits among those who may already enjoy advantages on the labor market.
- One key finding is that online platforms might increase the benefits of social networks for people who are already doing well in the job market.
- Further, our study also informs research on endogenous network formation (e.g. Jackson 2010), which often rests on the assumption of homogenous agents.
- Our study also adds to research on how networks form naturally, which usually assumes that all people are similar.
- Among the few exceptions is Song, and van der Schaar (2015) who model network formation under complete information suggest that stable links are created with agents of relatively high value only which is broadly consistent with our findings of such agents to be members of online networks.
- One exception is a study by Song and van der Schaar, which shows that networks form between valuable people, supporting our finding that successful individuals join online networks.
- The paper proceeds as follows. In Section 2, we review the literature and develop our hypotheses. Subsequently, we describe the data set in Section 3. Section 4 contains our empirical results. Finally, Section 5 concludes the paper.
- The rest of our paper is organized like this: Section 2 reviews other studies and our hypotheses, Section 3 describes our data, Section 4 shows our results, and Section 5 concludes the paper.
Explanation Line by Line in Simple Language:
- In the following section, we hypothesise about the drivers of professional online network membership.
- In this section, we will suggest reasons why people join professional online networks.
- We will first consider arguments which suggest that individuals positively select into professional SNSs, followed by a review of arguments in favour of adverse selection.
- First, we will look at reasons why successful people join these networks, then we will consider reasons why less successful people might join.
- Finally, we propose that the environment an individual is exposed to that influences the potential for social interaction increases the likelihood of professional SNS membership.
- Finally, we suggest that the social environment a person is in can make them more likely to join a professional network.
Positive Selection into Professional SNS
- A simple registration process is sufficient to join a social online network, and there are little direct setup costs and typically, there are no recurring fees at least for a non-premium membership.
- It’s easy to join a professional network online, with no big costs and usually no fees for basic membership.
- However, in practice, one of the main limitations seems to be lack of awareness or a lack of recognition of the value of the service the new online platform provides, given that the main point of entrance is by invitation of existing members (Katona, Zubcsek, and Sarvary 2011).
- But in reality, many people don’t join because they don’t know about the network or don’t see its value, and most join through invitations from current members.
- Thus, the likelihood that an individual joins a professional SNS should depend on whether she entertains relationships with active platform members who are motivated to invite her.
- So, whether someone joins often depends on if they have connections with current members who want to invite them.
- While members may just invite friends they like best, it is plausible to assume that many invitations are driven by the desire to benefit from the improved access to the invited individual.
- Members might invite their friends, but often they invite people they think will be useful to know professionally.
- Hence, the value that an individual provides to others when joining their SNS should increase the likelihood to receive an invitation.
- Therefore, if someone can offer value to others, they are more likely to be invited to join.
- While there are no studies formally modelling membership dynamics at online platforms, similar intuitions as in models of endogenous network formation under complete information suggest that stable links are created primarily with agents of relatively high value (e.g. Song and van der Schaar 2015).
- Although there are no specific studies on how people join these platforms, it’s logical to think that valuable people form strong connections, based on existing theories.
- Indeed, Campbell, Marsden, and Hurlbert (1986) have documented that individuals tend to be more motivated to form relationships with others if they are able to generate certain personal benefits in the future.
- Research has shown that people are more likely to form relationships if they see future benefits.
- Relatedly, Snidjers (1999, 1334) showed that individuals become important actors in social networks when others can “successfully draw on the resources controlled by their relational partners.”
- Another study found that people become key players in networks when others can benefit from their resources.
- In the professional context, such benefits could include the provision of resources that individuals support on their work, e.g. through increasing their job performance, which may ultimately lead to a promotion or a move to an otherwise more attractive position (Wolff and Moser 2009; Lin, Ensel, and Vaughn 1981).
- In a professional setting, these benefits might be help with work that leads to better job performance, promotions, or more attractive job offers.
- The resource that is likely to generate substantial support to others is an individual’s level of education which is the basis for advice and problem solving capacity.
- A key resource people can offer is their education, which helps with advice and problem-solving.
- Thus, we arrive at our first hypothesis:
- So, our first guess is:
- Hypothesis 1a: Individuals with higher levels of education are more likely to join professional SNS.
- Hypothesis 1a: People with higher education levels are more likely to join professional online networks.
- Another important source of career support that may render individuals attractive targets on professional SNS is their position as gatekeepers to prestigious or otherwise desirable jobs.
- Another reason people might join is if they have jobs that control access to desirable positions.
- Given that about job vacancies is decided typically at elevated levels in the organizational hierarchy, we propose the following hypothesis:
- Since job openings are often decided by higher-ups, we suggest the next guess:
- Hypothesis 1b: Managerial employees at higher levels of the organization are more likely to join professional SNS.
- Hypothesis 1b: Managers and higher-level employees are more likely to join professional online networks.
Explanation Line by Line in Simple Language:
Adverse Selection and Professional SNS Membership
- Previously, we hypothesized about a positive selection into professional online networks, based on the expectation that value to others gives rise to a higher propensity to get invited to join the platform.
- Earlier, we suggested that successful people are more likely to join professional online networks because they are valuable to others.
- An alternative view suggests that professional SNS suffer from adverse selection.
- Another perspective is that professional networks may attract people who are struggling in their careers.
- The basic idea is that professional SNS provide career-related benefits, and individuals who are dissatisfied about their career status may select into these networks, anticipating that a membership would help them to improve their career outcome.
- The main idea is that people who are unhappy with their careers might join professional networks hoping to improve their situation.
- One of the obvious career-related benefits professional SNS may provide is to support informal job search, that is, job matching that is mediated by members of the individual’s social network (Calvó-Armengol and Jackson 2007).
- One clear benefit of professional networks is helping with job searches through connections.
- Matching individuals to vacant jobs is notoriously plagued by search frictions (Stigler 1962; Mortensen 1970; Mortensen and Pissarides 1994), and social networks partially alleviate these frictions through better access to information about vacancies and through solving for other problems of asymmetric information (see surveys in Ioannides, and Loury 2004; Topa 2011; Galenianos 2014).
- Finding the right job is often difficult due to various issues, but social networks help by providing better information and reducing other problems.
- One of the important insights from the social network literature is the difference between the value of so-called strong and weak ties.
- Social network research highlights the difference between strong and weak connections.
- While strong ties refer to relationships characterized by intense and frequent information exchange, weak ties are typically associated with an individual’s network periphery that give rise to a low intensity of information exchange (Granovetter 1973).
- Strong ties are close relationships with frequent communication, while weak ties are more distant connections with less communication.
- Perhaps surprisingly, weak ties (or the network periphery) seem to be specifically important to obtain valuable vacancy-related information (e.g. Granovetter 1973, 1983), perhaps because these sources provide information to the individual that is useful because it would be unlikely to reach her through alternative channels.
- Surprisingly, weak ties are crucial for finding job information, as they can provide unique information not available through close connections.
- While communication between both weak and strong ties may migrate from other communication technologies to the professional SNS, we argue that professional online networks hold an advantage when it comes to communication between weak ties relative to strong ties.
- While both types of connections might move to professional networks, these networks are especially good for communicating with weak ties.
- As mentioned, strong ties are characterised by a high frequency of information exchange even absent social online platforms. Hence, the flow of specific career-relevant information is less likely to be improved through LinkedIn and similar networks.
- Strong ties already have frequent communication, so professional networks don’t significantly improve their information exchange.
- In contrast, professional SNS enable individuals to manage and communicate within larger networks. Hence, they provide for opportunities to generate relationships that would not have been formed in the absence of those platforms.
- Professional networks help people manage larger networks and form new connections that wouldn’t exist otherwise.
- Moreover, LinkedIn, Xing and similar network sites enable users to more easily communicate with one’s network periphery (Bakshy, et al. 2012).
- Sites like LinkedIn and Xing make it easier to communicate with distant connections.
- Thus, we expect that professional SNS help their members to primarily gain career-related information advantages through weak-tie effects, which may ultimately materialise in tangible career outcomes.
- Therefore, we believe that professional networks help users gain valuable job information through weak ties, leading to better career opportunities.
- Furthermore, professional SNS may also facilitate the search process for employers by making use of the social relationships of their current employees.
- Professional networks can also help employers find candidates by using the connections of their current employees.
- Firms have already previously relied on the referrals by current employees as a recruitment device (Saloner, 1985; Montgomery 1991).
- Companies already use employee referrals for hiring.
- Platforms such as LinkedIn enable firms to systematically use the contacts of their employees to get candidates referred to open positions, allowing firms to use it as a screening mechanism to select high-ability workers (Ioannides and Loury 2004).
- LinkedIn and similar platforms help companies systematically use employee contacts to find high-quality candidates.
- As a result, individuals who are unsatisfied with their current career status might be more likely to join professional SNSs because may expect to obtain more outside offers and to benefit from improved job matches.
- People unhappy with their careers might join professional networks expecting to get better job offers and matches.
- There are a number of measures to identify individuals who may perceive a gap between their career aspirations and the status quo.
- There are several ways to identify people who feel there is a gap between their career goals and their current situation.
- The first one we use is pay satisfaction since pay is a central career outcome (Gazioglu and Tansel 2006).
- The first measure is satisfaction with pay, as it is a key career outcome.
- The second measure is residual pay which is defined as the residual from a regression of log pay on a number of wage determinants such as education, gender, industry, firm size, and age (Nandi and Nicoletti 2014).
- The second measure is residual pay, calculated as the difference between actual pay and expected pay based on factors like education, gender, industry, and age.
- Residual pay is a more objective measure of relative career outcome up to non-observable factors.
- Residual pay is a more objective measure of career success compared to non-measurable factors.
- Finally, we consider the relative changes of pay an individual expects to experience over the next five years.
- Finally, we look at how much people expect their pay to change in the next five years.
- The lower are these expectations, the more inclined an individual should be to join the professional platform under the adverse selection hypothesis.
- If people expect low pay increases, they are more likely to join professional networks according to the adverse selection hypothesis.
- Hence, we obtain:
- Therefore, we propose:
- Hypothesis 2a: Individuals with lower levels of pay satisfaction are more likely to join professional SNSs.
- Hypothesis 2a: People who are less satisfied with their pay are more likely to join professional networks.
- Hypothesis 2b: Individuals with lower levels of residual pay are more likely to join professional SNSs.
- Hypothesis 2b: People with lower residual pay are more likely to join professional networks.
- Hypothesis 2c: Individuals with lower expectations about their wage changes in the following five years are more likely to join professional SNSs.
- Hypothesis 2c: People with lower expectations for pay increases in the next five years are more likely to join professional networks.
- Individuals may for various reasons be unsatisfied with their current career situation, and our list of measures (and drivers) of adverse selection cannot be exhaustive.
- There are many reasons why people might be unhappy with their careers, and our list of factors for adverse selection isn’t complete.
- For example, specific constellations in the organisation that limits career progress could make individuals to adversely select into professional SNS.
- For instance, organizational factors that limit career growth might push people to join professional networks.
- However, one very prominent factor that previous research has identified as a structural obstacle for certain individuals such as members of minorities to advance in their careers or be paid wages one would view as appropriate is associated with discriminatory behaviour of employers.
- One well-known factor is employer discrimination, which prevents minorities and women from advancing or getting fair pay.
- The literature on “glass ceilings” specifically shows that female employees face such problems (e.g. Blau and Kahn 2000; Sabatier 2010).
- Research on “glass ceilings” shows that women face these issues.
- Professional SNS may be used by women as a means to alleviate problems of discriminatory employment practices.
- Women might use professional networks to overcome discriminatory practices.
- Hence, we obtain the following hypothesis:
- Therefore, we suggest the following hypothesis:
- Hypothesis 2d: Women are more likely to join professional SNSs.
- Hypothesis 2d: Women are more likely to join professional networks.
The Social Environment and Professional SNS Membership
Introduction
“In the following, we change the perspective from the individuals’ motivation to join professional SNS to environmental factors that may affect this decision.”
- This section shifts the focus from personal reasons for joining professional social networking sites (SNS) to external factors that could influence this decision.
“Specifically, we focus on geographic and organizational conditions associated with the potential number of offline contacts to explain different network adoption rates.”
- We will look at how geographic (location-based) and organizational (workplace-based) conditions impact the likelihood of people joining professional networks.
Geographic Factors
“First, we argue that individuals living in more populated areas are more likely to establish social professional relationships.”
- People in densely populated areas are more likely to form professional connections.
“This holds because first, the number of random interactions mechanically increases with the size of the population (Glaeser 1999).”
- In larger populations, there are more opportunities for random social interactions simply because there are more people around.
“Secondly, employees in more populated areas tend to be more engaged in more communication across organizations, for example, when local clusters in the same region inhabit suppliers and their customers (Charlot and Duranton 2006).”
- In populous areas, employees often communicate more across different organizations, such as between suppliers and customers within the same region.
“Moreover, epidemiological models suggest that social interaction increases as cities grow bigger, which are supported by empirical evidence (Schläpfer et al. 2014).”
- Models and studies show that social interactions increase as cities get larger, supported by data and research.
“Finally, more populated areas should also, on average, give rise to more relevant contacts.”
- Densely populated areas tend to offer more opportunities to meet people who can be valuable professional contacts.
“Thus, given that the likelihood to get invited to a professional SNS, all else equal, should rise with the number of potential professional contacts, we expect city size (as an indicator for how populated an area is) to be associated with a higher likelihood to be a member of a professional SNS.”
- Therefore, the chance of being invited to join a professional SNS increases with the number of potential professional contacts in a city. We predict that city size is linked to a higher likelihood of joining a professional SNS.
Organizational Factors
“The working environment represents another constraint on how individuals interact.”
- The workplace environment also affects how people interact.
“Larger organizations naturally provide more opportunities for social exchange.”
- Bigger companies offer more chances for social interactions among employees.
“First, given that complexity of operations tends to increase with the size of the organization, larger companies implement a higher degree of the division of labor and an increased specialization of skills and functions (Daft 2012; Baron and Bielby 1986), which give rise to a higher demand to coordinate and communicate with other employees.”
- Larger organizations have more complex operations, requiring more division of labor and specialized roles, leading to a greater need for coordination and communication among employees.
“Second, even though employees working in large firms might be spread over different locations, typically, larger firms concentrate their employees in bigger units than smaller firms (Mellow 1982).”
- Although employees in large firms might be spread out geographically, these firms generally group their employees into larger units compared to smaller companies.
“Hence, the pool of potential contacts increases with the size of the employing organization, and we expect individuals working at larger firms to be more likely to receive an invitation to join a professional SNS.”
- Therefore, the number of potential professional contacts grows with the size of the organization, making it more likely for employees in larger firms to be invited to join a professional SNS.
Adverse Selection Considerations
“On the other hand, individuals who are hampered by a lack of opportunities to form professional connections may be more motivated to use their membership at professional SNS to overcome these disadvantages.”
- Conversely, people with fewer chances to make professional connections might be more motivated to join professional SNS to mitigate these disadvantages.
“Hence, one may expect adverse selection along these dimensions as well.”
- This suggests that there could also be adverse selection, where those with fewer opportunities might disproportionately join these networks.
“Moreover, the effect of organization size on the number of potential professional contacts might be somewhat muted by the fact that employees at SMEs need more strongly to cooperate across firm boundaries to access necessary resources for firm success (Lipparini and Sobrero 1994).”
- The impact of organization size on potential contacts may be less significant because employees in small and medium-sized enterprises (SMEs) often need to collaborate more across company boundaries to succeed.
“Ultimately, it is an empirical question whether offline and online networks serve as complements or substitutes.”
- Whether offline and online networks complement or substitute each other is a question that requires empirical research.
“As a result of the arguments presented above, we obtain the following two hypotheses:”
- Based on the above arguments, we propose two hypotheses:
“Hypothesis 3a: Individuals living in densely populated areas are more likely to join a professional SNS.”
- People in densely populated areas are more likely to join professional SNS.
“Hypothesis 3b: Individuals working at larger organizations are more likely to join a professional SNS.”
- Employees at larger organizations are more likely to join professional SNS.
Data
Introduction
“We use a large data set that is based on two rounds of internet-based surveys conducted on three websites in 2009 and 2011.”
- The study uses data from two rounds of surveys conducted online in 2009 and 2011.
“The first two of these are the websites of the German weekly magazines Der Spiegel (http://www.spiegel.de, also referred to as Spiegel Online) and Manager Magazin (http://www.manager-magazin.de). The third one is the website of the professional SNS Xing.”
- The surveys were conducted on the websites of Der Spiegel, Manager Magazin, and the professional social networking site Xing.
“For a few days during 2009 and 2011, visitors of these websites were invited to participate in the survey.”
- During certain days in 2009 and 2011, visitors to these websites were asked to participate in the survey.
“Participation was not remunerated, but after filling in the survey, every participant received an analysis comparing their own compensation to the typical pay of an individual from the same industry performing a similar job.”
- Participants were not paid, but they received a personalized compensation report after completing the survey.
“Hence, the survey was incentive-compatible in the sense that only truthful reporting yielded a useful feedback.”
- The incentive for truthful reporting was that only accurate information would result in a useful compensation comparison.
“Survey participants were asked to provide information on a number of job-related issues such as pay and pay satisfaction, employer size, and industry.”
- Participants provided information on their salary, job satisfaction, employer size, and industry.
“Further, participants were requested to disclose information on the location of residence, education, and membership at private and professional online networks.”
- They also shared details about their place of residence, education, and membership in private and professional online networks.
“In total, the database is composed of 251,693 observations.”
- The dataset contains a total of 251,693 survey responses.
Data Cleaning
“Given our research question, we had to ignore all observations sourced from xing.com.”
- The study focuses only on responses from the Der Spiegel and Manager Magazin websites, excluding data from Xing.
“Moreover, we also dismissed observations from the online platform of Das Manager Magazin because of the very selected readership of this magazine.”
- Responses from Manager Magazin were also excluded due to its highly selective readership.
“We exclusively analysed the survey responses from Spiegel Online, which is the most widely read German-language news website.”
- The analysis was confined to responses from Spiegel Online, the most popular German-language news site.
“Non-response bias might be a serious concern, because one of the incentives to participate in the survey was to receive the individualized compensation report.”
- There is a concern about non-response bias because the compensation report might have influenced who chose to participate.
“Since we are provided with demographic information of the regular readership of this online magazine, we are able to address the selectivity associated with survey participation (see below).”
- The study addresses potential bias by comparing the survey sample with the demographic information of regular Spiegel Online readers.
“Once we pick Spiegel Online entries, we are left with 95,082 data points.”
- After filtering for Spiegel Online responses, 95,082 data points remain.
“After removing observations that are not from full-time private sector employees and after missing data is dropped, our sample is reduced to 71,335 data points.”
- Further filtering to include only full-time private sector employees and removing incomplete data results in 71,335 data points.
Data Summary
“A summary of the data and an overview of the realizations of some of the variables of interest across the different social online networks are displayed in Table 1.”
- Table 1 provides a summary and key statistics of the dataset.
“Average values for our sample are reported in column (1). Survey participants earned around 60,000 EUR on average as gross salaries, indicating that the sample is biased towards individuals with higher-than-average incomes in Germany.”
- The average salary in the sample is around 60,000 EUR, suggesting a bias towards higher-income individuals.
“The second row shows the average level of pay satisfaction which is a dichotomous variable. The sample is about equally split between the two possible responses.”
- Pay satisfaction, measured as a yes/no variable, is nearly evenly split among respondents.
“Comparing this value to estimates of pay satisfaction in comparable studies (e.g. Cloutier, Morin, and Renaud 2013), it seems that our sample does slightly overrepresent individuals who tend to be less satisfied with their pay.”
- The sample slightly overrepresents individuals who are less satisfied with their pay compared to other studies.
“One remarkable feature is that only around one seventh of the entire sample is female (third row). However, below, we find that a large part of this gender gap is due to the overrepresentation of men in the population from which the survey is sampled.”
- Only about 1/7th of respondents are female, largely due to an overrepresentation of men in the website’s regular readership.
“In Table 1, we look more closely at differences in wages and other variables across professional SNS (LinkedIn and Xing) and private SNS (Facebook, Wer-kennt-wen, MeinVZ and Lokalisten).”
- Table 1 compares wages and other variables between professional and private social networks.
“First note that Xing (49%) and Facebook (35%) are the most popular networks in our sample. Only around 11% of the survey participants are registered LinkedIn members.”
- Xing and Facebook are the most popular networks among respondents, with LinkedIn having only 11% membership.
“The other private networks cover between 3% and 35% of the survey participants.”
- Membership in other private networks ranges from 3% to 35%.
“Regarding compensation and pay satisfaction, individuals registered with Xing or LinkedIn receive much higher salaries and are on average more satisfied with their pay than average numbers suggest for members of private social networks.”
- Xing and LinkedIn members have higher salaries and greater pay satisfaction compared to private social network members.
“LinkedIn members receive the highest average salary levels and report the highest levels of pay satisfaction.”
- LinkedIn members have the highest average salaries and pay satisfaction.
“In our sample, women are slightly less likely to be a member of a professional than a private SNS.”
- Women in the sample are less likely to join professional SNS compared to private ones.
“Table 1 also shows the distribution of online network membership across some selected industries.”
- Table 1 includes data on network membership by industry.
“There are marked differences in the usage of professional SNS across industries. Perhaps not surprisingly, employees in the Consulting, IT, and Telecommunication industries have much higher membership rates than other industries.”
- Professional SNS membership rates vary significantly by industry, with Consulting, IT, and Telecommunications showing higher rates.
“Similar distributions emerge for private online networks.”
- Similar patterns are seen for private SNS membership.
“Considering educational outcomes, Table 1 shows that the average member at LinkedIn and Xing is more likely to possess a higher degree such as a university degree, a PhD or a MBA degree than the average user at private SNS.”
- LinkedIn and Xing members are more likely to have advanced degrees compared to private SNS members.
“Finally, we find that employees at larger firms are more likely to be a member at a professional SNS, which does not hold for members of a private social online network.”
- Employees at larger firms are more likely to join professional SNS, but this pattern does not hold for private SNS.
“In contrast, membership at all social networks is strongly and positively associated with city size.”
- Membership in both professional and private SNS is positively correlated with living in larger cities.
Addressing Survey Response Bias
“In order to examine the extent of a potential survey response bias, let us compare individuals in our sample with the regular readership of Spiegel Online (see Table 2).”
- The study compares survey respondents to regular Spiegel Online readers to check for response bias.
“We find substantial discrepancies between the incomes of survey participants and regular readers.”
- There are significant income differences between survey participants and regular readers.
“Moreover, the sample over-represents individuals between 31 and 50 years of age and those with a degree from a technical college or a university.”
- The sample has more individuals aged 31-50 and those with higher education degrees than the general readership.
“Finally, Table 2 shows that the sample is biased towards big-city residents.”
- The sample has a higher proportion of big-city residents compared to the regular readership.
“Our empirical analysis will take account of the bias in responses.”
- The study will account for these biases in its analysis.

Sure, let’s break down the table step by step to make it clear and easy to understand. We will go through each row and column with detailed explanations.
Table Overview
The table provides a summary of data about participants who use professional and private social networks. The data includes information about their pay, pay satisfaction, gender distribution, industry, firm size, city size, and education level.
Columns
- Sample: This is the general data for all participants.
- LinkedIn: Data for participants who use LinkedIn.
- Xing: Data for participants who use Xing.
- Facebook: Data for participants who use Facebook.
- Wer-kennt-wen: Data for participants who use Wer-kennt-wen.
- MeinVZ: Data for participants who use MeinVZ.
- Lokalisten: Data for participants who use Lokalisten.
Rows
- Pay: The average yearly gross salary in thousand Euros.
The phrase “The average yearly gross salary in thousand Euros” means the average amount of money that people earn in one year before any taxes or deductions, expressed in thousands of Euros.
Let’s break it down:
- Average: This is the mean value, calculated by adding up all the salaries and then dividing by the number of people.
- Yearly: This refers to the amount of money earned over the course of one year.
- Gross Salary: This is the total amount of money earned before any deductions such as taxes, social security, or other withholdings are taken out.
- In Thousand Euros: Instead of showing the full number, the salary is divided by 1,000 to simplify the figures. For example, if the salary is 60.01 thousand Euros, it means 60,010 Euros.
Example:
If a table shows an average yearly gross salary of 60.01 thousand Euros, it means that on average, people earn 60,010 Euros per year before any taxes or deductions are taken out.
- Pay Satisfaction: The percentage of participants who are satisfied with their pay.
Pay Satisfaction refers to the percentage of survey participants who reported being satisfied with their pay. It is a measure of how happy people are with the amount of money they earn.
Example Explanation:
- Imagine a survey was conducted among 100 people about their job satisfaction.
- One of the questions asked participants if they were satisfied with their pay, and they could answer “yes” or “no.”
- If 49 out of these 100 people said they were satisfied with their pay, then the pay satisfaction percentage would be 49%.
In the table:
- Column Header: Pay Satisfaction – This column shows the percentage of participants who reported being satisfied with their pay.
- Values:
- For LinkedIn users, the pay satisfaction percentage is 55.06%. This means that 55.06% of LinkedIn users in the survey reported that they were satisfied with their pay.
- For Xing users, it is 49.76%. This means 49.76% of Xing users in the survey were satisfied with their pay.
- For Facebook users, it is 48.15%. This means 48.15% of Facebook users in the survey were satisfied with their pay.
Detailed Example:
- Professional Network (LinkedIn):
- If 100 LinkedIn users participated in the survey, 55.06% being satisfied means about 55 of these users said “yes” when asked if they were satisfied with their pay.
- Private Network (Facebook):
- If 100 Facebook users participated in the survey, 48.15% being satisfied means about 48 of these users said “yes” when asked if they were satisfied with their pay.
Interpretation:
- Higher percentages indicate a greater proportion of participants satisfied with their pay.
- Comparing these percentages helps understand which group of users, on average, is more satisfied with their earnings. For instance, LinkedIn users appear more satisfied with their pay compared to Facebook users according to the survey data.
- Female: The percentage of female participants.
- Industry (selected): Distribution of participants across various industries.
Industry (selected): Distribution of participants across various industries indicates how survey participants are spread across different industries. This helps to understand which industries the participants work in and how their distribution compares across different social networks.
Explanation with Examples:
- Column Header: Industry (selected) – This shows the percentage of participants from various industries who use professional and private social networks.
- Values:
- For LinkedIn users in the Automotive industry, the percentage is 7.17%. This means that 7.17% of LinkedIn users in the survey work in the Automotive industry.
- For Facebook users in the Automotive industry, the percentage is 30.45%. This means that 30.45% of Facebook users in the survey work in the Automotive industry.
Detailed Example:
- Professional Network (LinkedIn):
- Automotive: If there are 100 LinkedIn users surveyed, 7.17% working in the Automotive industry means about 7 of these users work in the Automotive industry.
- Consulting: If there are 100 LinkedIn users surveyed, 22.43% working in the Consulting industry means about 22 of these users work in the Consulting industry.
- Private Network (Facebook):
- Automotive: If there are 100 Facebook users surveyed, 30.45% working in the Automotive industry means about 30 of these users work in the Automotive industry.
- Consulting: If there are 100 Facebook users surveyed, 49.41% working in the Consulting industry means about 49 of these users work in the Consulting industry.
Comparing Industries:
- Automotive Industry:
- LinkedIn: 7.17%
- Facebook: 30.45%
- This shows that a smaller percentage of LinkedIn users work in the Automotive industry compared to Facebook users.
- Consulting Industry:
- LinkedIn: 22.43%
- Facebook: 49.41%
- This shows that a smaller percentage of LinkedIn users work in the Consulting industry compared to Facebook users.
Interpretation:
- Higher percentages indicate a greater proportion of participants from that industry using the network.
- The distribution helps understand industry representation within different social networks. For instance, the Consulting industry has a higher representation on LinkedIn compared to the Automotive industry.
By analyzing these percentages, one can gain insights into which industries are more prevalent among users of professional networks (like LinkedIn) compared to private networks (like Facebook). This information can be useful for targeted marketing, networking strategies, or understanding industry-specific trends in social media usage.
- Firm size (sales): Distribution based on the size of the firm measured by sales in Euros.
- City size: Distribution based on the size of the city where participants live.
- Highest Education: Distribution based on the highest level of education attained by participants.
Detailed Explanation
Let’s go through each section of the table with examples:
Pay (Yearly Gross Salary in Thousand Euros)
- Sample: The average pay for all participants is 60.01 thousand Euros.
- LinkedIn: Participants using LinkedIn have a higher average pay of 79.93 thousand Euros.
- Xing: Participants using Xing have an average pay of 61.74 thousand Euros.
- Facebook: Participants using Facebook earn an average of 56.74 thousand Euros.
- Wer-kennt-wen: Participants earn an average of 54.84 thousand Euros.
- MeinVZ: Participants earn an average of 44.94 thousand Euros.
- Lokalisten: Participants earn an average of 53.55 thousand Euros.
Example: If you are a participant using LinkedIn, your average yearly pay is higher compared to someone using Facebook or Wer-kennt-wen.
Pay Satisfaction (Percentage)
- Sample: 49.07% of all participants are satisfied with their pay.
- LinkedIn: 55.06% of LinkedIn users are satisfied with their pay.
- Xing: 49.76% of Xing users are satisfied with their pay.
- Facebook: 48.15% of Facebook users are satisfied with their pay.
- Wer-kennt-wen: 47.21% of Wer-kennt-wen users are satisfied.
- MeinVZ: 47.75% of MeinVZ users are satisfied.
- Lokalisten: 46.77% of Lokalisten users are satisfied.
Example: If you use LinkedIn, you are more likely to be satisfied with your pay compared to users of other networks like Facebook or MeinVZ.
Female (Percentage)
- Sample: 15.90% of all participants are female.
- LinkedIn: 14.30% of LinkedIn users are female.
- Xing: 15.80% of Xing users are female.
- Facebook: 19.27% of Facebook users are female.
- Wer-kennt-wen: 17.49% of Wer-kennt-wen users are female.
- MeinVZ: 18.42% of MeinVZ users are female.
- Lokalisten: 16.48% of Lokalisten users are female.
Example: Facebook has a higher percentage of female users (19.27%) compared to LinkedIn (14.30%).
Industry (Selected)
- This section shows the distribution of participants in different industries, with percentages indicating the proportion within each social network.
Example:
- In the Automotive industry:
- LinkedIn: 7.17% of LinkedIn users work in Automotive.
- Xing: 47.09% of Xing users work in Automotive.
- Facebook: 30.45% of Facebook users work in Automotive.
Firm Size (Sales)
- This section indicates the distribution of participants based on the size of their firm measured by sales.
Example:
- Firms with sales less than 1 million Euros:
- LinkedIn: 7.27% of LinkedIn users work in these firms.
- Xing: 41.12% of Xing users work in these firms.
- Facebook: 36.64% of Facebook users work in these firms.
City Size
- This section indicates the distribution of participants based on the size of the city where they live.
Example:
- In cities with populations less than 50,000:
- LinkedIn: 7.18% of LinkedIn users live in these cities.
- Xing: 41.36% of Xing users live in these cities.
- Facebook: 26.71% of Facebook users live in these cities.
Highest Education
- This section shows the distribution of participants based on their highest level of education.
Example:
- University degree:
- LinkedIn: 14.54% of LinkedIn users have a university degree.
- Xing: 55.49% of Xing users have a university degree.
- Facebook: 38.11% of Facebook users have a university degree.
Summary
The table provides a detailed comparison of participants using different social networks, focusing on various aspects like pay, pay satisfaction, gender, industry, firm size, city size, and education. It highlights how users of professional networks like LinkedIn and Xing generally have higher pay, higher pay satisfaction, and higher educational qualifications compared to users of private networks like Facebook and Wer-kennt-wen.
Sure, let’s break down each line of the passage for better understanding:
- “In the following, we report our empirical results.”
- This means that the next section will present the results obtained from their empirical analysis (i.e., results based on observation or experiment rather than theory).
- “In order to test our hypotheses, we estimate the following Probit model:”
- To test the assumptions or predictions (hypotheses) they have made, they use a statistical model called the Probit model. They will describe this model in the next line.
- “Networkit = β1 + β2xit + 𝜖it (1)”
- This is the equation of the Probit model they are using.
- Networkit represents the dependent variable, which in this case is whether the individual i is a member of a professional network (either LinkedIn or Xing) in year t.
- β1 is the intercept term, a constant that represents the baseline probability of network membership.
- β2xit is the term representing the influence of various independent variables (xit) on the likelihood of network membership. β2 is a vector of coefficients that measure the impact of each variable in xit.
- 𝜖it is the error term, capturing the variability in network membership that isn’t explained by the model.
- “where Network is either LinkedIn or Xing.”
- The dependent variable, Network, can either be membership in LinkedIn or Xing.
- “Subscripts i and t denote the individual and the year of observation.”
- The subscript i refers to different individuals, and t refers to different years.
- “Vector x encompasses the variables that we expect to drive network membership,”
- The vector x includes all the independent variables (or factors) they believe will influence whether someone joins LinkedIn or Xing.
- “𝜖 is the error term.”
- The term 𝜖 is the error term in the model, which accounts for any variation in network membership not explained by the variables in x.
- “We estimate (1) as a Probit model through a Maximum Likelihood approach (Wooldridge 2010).”
- They are using a statistical technique called the Probit model to estimate the equation (1).
- They use the Maximum Likelihood Estimation (MLE) method to find the best-fitting model, as suggested by Wooldridge (2010), a reference to a textbook or paper explaining this methodology.
Example for clarity:
Imagine you want to predict whether students in a school join a sports club (LinkedIn or Xing in this case). Your hypothesis might be that factors like age, grade, and interest in sports (these are the x variables) influence their decision. The Probit model helps you understand the relationship between these factors and the likelihood of joining the sports club. The error term (𝜖) captures any other reasons not included in your model that might affect a student’s decision. Using MLE helps ensure your predictions are as accurate as possible based on the data you have.
A Probit model is a type of regression used in statistics to model binary outcome variables. It is particularly useful when the dependent variable is a binary outcome (i.e., it has only two possible values, typically coded as 0 and 1). The Probit model estimates the probability that a particular event occurs based on one or more predictor variables.
Key Concepts of the Probit Model:
- Binary Dependent Variable:
- The outcome variable in a Probit model is binary. For example, it could be whether an individual joins a social network (yes=1, no=0).
- Latent Variable:
- The Probit model assumes the existence of an underlying continuous latent variable (Y*) that determines the observed binary outcome.
- This latent variable can be thought of as the propensity or inclination towards the event occurring.
- Link Function:
- The relationship between the latent variable (Y*) and the observed outcome (Y) is modeled using the cumulative distribution function (CDF) of the standard normal distribution.
- The Probit model uses the inverse of the standard normal CDF (also known as the probit function) to transform the linear combination of predictors into a probability.
Summary:
- The Probit model is used for binary dependent variables.
- It models the probability of an event occurring based on predictor variables.
- It uses the cumulative normal distribution to link the predictors to the probability of the outcome.
- It’s particularly useful when the outcome is influenced by a latent variable that follows a normal distribution.